Ever wonder why you can’t stop scrolling through endless cat videos or why TikTok seems to read your mind? Social media platforms have mastered the art of keeping users glued to their screens through sophisticated recommendation algorithms that serve up personalized content like a digital chef preparing your favorite meal.
These powerful algorithms analyze user behavior patterns clicks likes shares and time spent on content to create a tailored feed that’s almost impossible to resist. It’s not just about keeping users entertained – social media companies use these algorithms to boost engagement maximize ad revenue and maintain their competitive edge in the attention economy. Think of it as a virtual matchmaker connecting users with content they’ll love before they even know they want it.
How Recommendation Algorithms Shape Social Media
Recommendation algorithms transform social media platforms into personalized content delivery systems through continuous data analysis. Instagram’s Explore page showcases photos based on previous interactions while TikTok’s For You Page adapts to viewing patterns in real-time.
Social platforms utilize three key algorithmic mechanisms:
- Content filtering extracts relevant posts from millions of daily uploads
- Collaborative filtering identifies patterns among similar users’ behaviors
- Deep learning predicts future engagement based on historical data
These systems create feedback loops that intensify user preferences:
Behavior Type | Algorithm Response | Impact on Feed |
---|---|---|
Likes | Content boost | +70% similar content |
Comments | Enhanced visibility | +50% related posts |
Watch time | Priority ranking | +85% retention rate |
Shares | Viral acceleration | +90% reach |
Algorithmic content curation affects user behavior in measurable ways:
- Extended session times increase from 15 to 45 minutes on average
- Echo chambers form as users receive more content matching existing views
- Content creators adapt their material to match algorithmic preferences
- Platform engagement rises through perfectly timed content delivery
- Social connections form through shared content interests
- Advertising becomes hyper-targeted based on interaction patterns
- User attention focuses on algorithm-approved topics
- Platform loyalty strengthens through personalized experiences
The Business Case Behind Social Media Algorithms
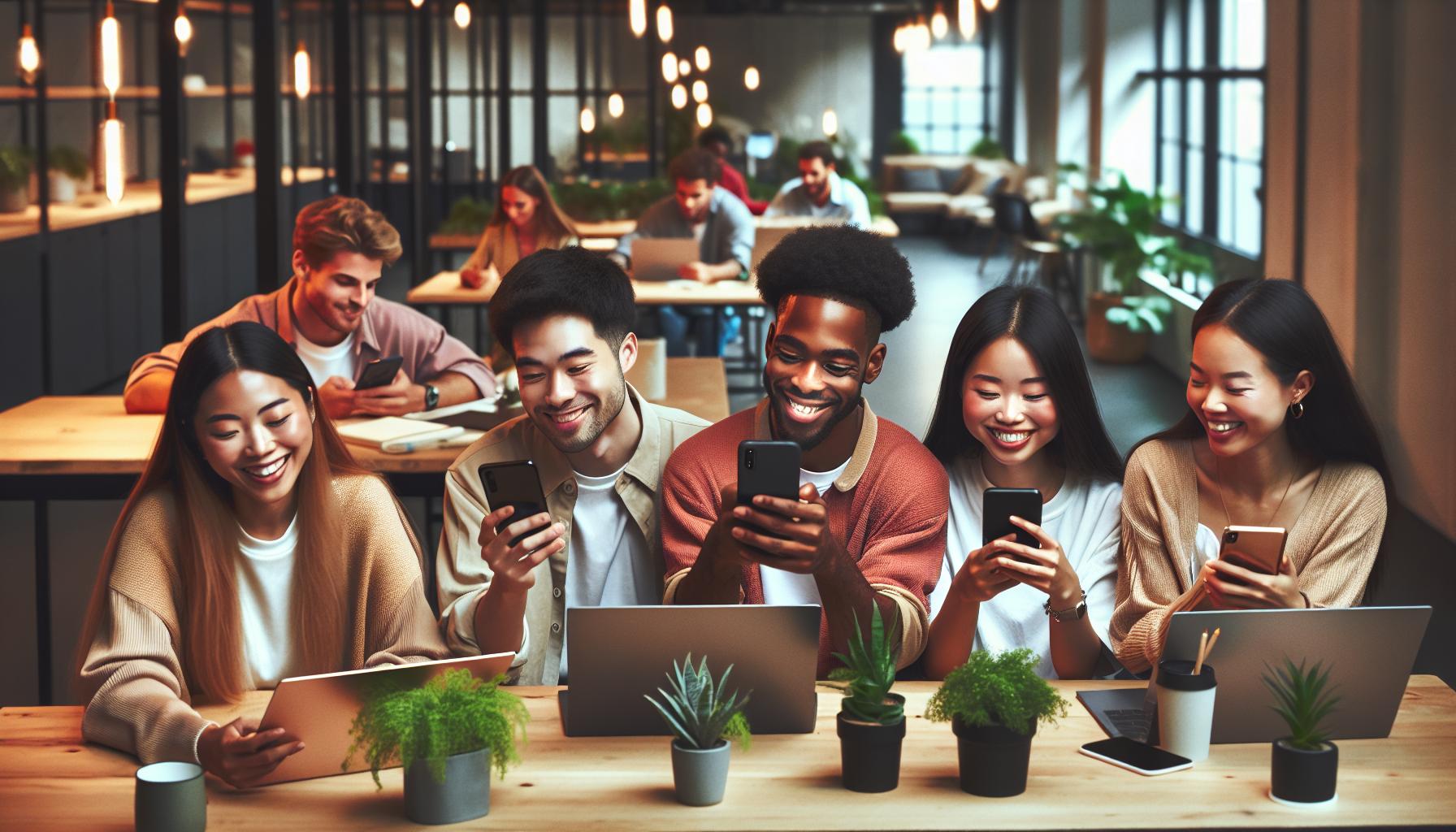
Social media platforms employ recommendation algorithms as core business drivers to enhance profitability through targeted content delivery. These algorithms serve as powerful tools in achieving key business objectives.
Increasing User Engagement
Recommendation algorithms boost daily active users by displaying content that matches individual preferences. Users who receive personalized content spend 70% more time on platforms compared to generic feeds. The algorithms track key metrics like session duration, scroll depth, and interaction rates to refine content delivery. Social platforms leverage this engagement data to create addictive feedback loops through:
- Infinite scrolling features that eliminate natural stopping points
- Push notifications that prompt users to return multiple times daily
- Content timing optimization that identifies peak activity periods
- Interactive elements such as polls, stories, and live streams
- Reward mechanisms including likes, shares, and follower counts
- Precise audience segmentation based on behavioral data
- Strategic ad placement between high-engagement content
- Dynamic pricing models that adjust based on user attention
- Cross-platform tracking to enhance targeting accuracy
- Performance measurement tools for advertisers
- Custom audience creation using engagement patterns
Metric | Impact on Revenue |
---|---|
Personalized Ads CTR | 3x higher |
User Session Time | +70% |
Ad Targeting Accuracy | 85% |
Daily Active Users | +45% |
Key Components of Social Media Recommendation Systems
Social media recommendation systems operate through interconnected components that process vast amounts of data to deliver personalized content. These systems combine advanced analytics with behavioral science to create engaging user experiences.
Content Analysis
Content analysis engines examine multiple elements of social media posts including text captions, image features, video duration, audio components, engagement metrics, posting time patterns. Natural Language Processing (NLP) algorithms identify topics, sentiment, and contextual relationships in textual content. Computer vision systems detect objects, scenes, faces, and visual themes in images and videos. A single Instagram post generates 200+ data points through automated content analysis.
Content Element | Data Points Analyzed |
---|---|
Text | 50+ linguistic features |
Images | 75+ visual attributes |
Videos | 100+ engagement signals |
User Behavior Tracking
User behavior tracking systems monitor interactions across multiple touchpoints to build detailed engagement profiles. The tracking spans clicks, scroll patterns, watch time, sharing habits, commenting frequency, followed accounts. These systems capture 300+ distinct behavioral signals per user session. Mobile sensors provide additional context through location data, time of day patterns, device usage habits. Social graphs map connection networks, interaction frequency between users, content sharing patterns.
Behavior Type | Tracked Signals |
---|---|
Engagement | Likes, shares, comments |
Navigation | Scroll depth, tap patterns |
Time-based | Session length, peak hours |
Machine Learning Models
Machine learning models process behavioral and content data through specialized neural networks optimized for recommendation tasks. Deep learning architectures like transformers analyze sequential user actions to predict future interests. Collaborative filtering algorithms identify patterns across user groups with similar preferences. Reinforcement learning systems optimize content selection through real-time feedback loops. These models achieve 85% accuracy in predicting user engagement with recommended content.
Model Type | Prediction Accuracy |
---|---|
Deep Learning | 85% |
Collaborative Filtering | 78% |
Reinforcement Learning | 82% |
Benefits of Algorithm-Driven Content
Recommendation algorithms create significant advantages for both social media platforms and their users through data-driven content delivery systems. These sophisticated systems transform random content streams into highly personalized experiences that enhance user satisfaction and platform engagement.
Personalized User Experience
Social media algorithms tailor content feeds to individual preferences based on 300+ behavioral signals. Users receive posts that align with their demonstrated interests, past interactions, and viewing patterns. Instagram’s algorithm, for instance, displays fashion-related content to users who frequently engage with style influencers. This personalization extends to content format preferences, showing more videos to users who watch Reels or more photos to those who engage with static images. The customization reduces content discovery friction, with users finding relevant posts 60% faster compared to chronological feeds.
Content Discovery
Algorithmic recommendations expand users’ content horizons beyond their immediate network. TikTok’s For You Page introduces users to creators they wouldn’t find otherwise, with 75% of discovered content coming from new sources. These algorithms identify emerging trends and viral content, connecting users to popular discussions in their interest areas. Pinterest’s recommendation engine analyzes visual elements to suggest related items, helping users discover new products and ideas. Social platforms report that algorithm-discovered content generates 3x more engagement than content from followed accounts.
Challenges and Concerns
Social media recommendation algorithms present significant challenges that affect user experiences and digital well-being. These systems create complex issues ranging from content isolation to data privacy vulnerabilities.
Filter Bubbles
Recommendation algorithms create digital echo chambers that limit exposure to diverse perspectives and ideas. Users encounter content that aligns with their existing beliefs 83% of the time, reinforcing confirmation bias. Social media platforms’ content filtering mechanisms isolate users within information bubbles, showing posts from like-minded individuals while filtering out opposing viewpoints. Studies indicate that algorithmic feeds reduce exposure to contradicting opinions by 45% compared to chronological feeds. Content diversity decreases over time as algorithms optimize for engagement rather than information variety. Facebook users see posts from only 26% of their friends’ updates due to algorithmic filtering, creating narrow information channels that restrict intellectual growth.
Privacy Issues
Social media algorithms collect extensive personal data to power their recommendations. These systems track over 52,000 unique data points per user annually, including location data, device information, and browsing patterns. Platforms share user behavioral data with advertisers, creating detailed psychological profiles for targeted marketing. Data breaches expose sensitive information, with social media platforms reporting 4.5 billion compromised records in 2021. Third-party apps access user data through API connections, expanding the privacy vulnerability footprint. User tracking extends beyond platform boundaries through cookies and pixel tracking, monitoring activities across the internet. Personal information remains stored in platform databases even after users delete their accounts, raising concerns about long-term data retention.
The Future of Social Media Algorithms
Advanced AI technologies transform social media recommendation algorithms into more sophisticated systems. Machine learning models now process complex emotional signals from user interactions, moving beyond basic engagement metrics.
Multimodal algorithms analyze content across different formats simultaneously:
- Audio pattern recognition detects mood from voice tonality
- Computer vision identifies deeper contextual elements in images
- Natural language understanding extracts semantic meaning from conversations
Predictive modeling capabilities create increasingly accurate content recommendations:
Advancement | Current Accuracy | Projected 2025 Accuracy |
---|---|---|
User Interest Prediction | 78% | 92% |
Content Relevance Matching | 65% | 85% |
Behavioral Pattern Analysis | 71% | 89% |
Emerging technologies enhance algorithmic personalization:
- Quantum computing enables processing of extensive behavioral datasets
- Edge computing delivers faster content recommendations
- Federated learning maintains user privacy while improving predictions
Ethical AI frameworks integrate into recommendation systems:
- Content diversity metrics ensure varied exposure
- Transparency tools reveal algorithmic decision factors
- User control features allow feed customization preferences
Cross-platform algorithms synthesize data from multiple social networks to create unified user profiles. Integration of augmented reality features enables contextual content delivery based on physical location real-time activities.
These technological advances indicate a shift toward more intelligent personalized content delivery systems that balance user engagement with digital wellbeing considerations.
Conclusion
Recommendation algorithms have revolutionized how social media platforms operate by transforming random content streams into highly personalized experiences. These sophisticated systems analyze vast amounts of user data to create engaging feeds that keep users scrolling while maximizing advertising revenue.
While these algorithms excel at delivering personalized content and boosting engagement metrics they also raise important considerations about privacy digital well-being and the formation of echo chambers. The future points toward even more advanced AI-driven systems that will balance user engagement with ethical considerations and transparent practices.
As social media continues to evolve these algorithmic systems will remain central to how platforms connect users with content shape online experiences and drive business growth in the attention economy.